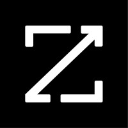
Sales intelligence software that identifies ideal prospects, builds custom contact lists, and provides real-time insights to help sales teams close more deals.
See more details See less details
With its advanced search filters, ZoomInfo allows users to identify decision-makers, track company news, and gain competitive intelligence. The platform also offers integrations with top CRMs for seamless data transfer and workflow automation.
Read our analysis about ZoomInfoBenefits of ZoomInfo
B2B Contact Data Accuracy With Hyper-Focused Segmentation
Seamless Integration With Your Workflow
Streaming Buyer Intent Data Tools
To ZoomInfo product page